Funding public goods using the Nash product rule
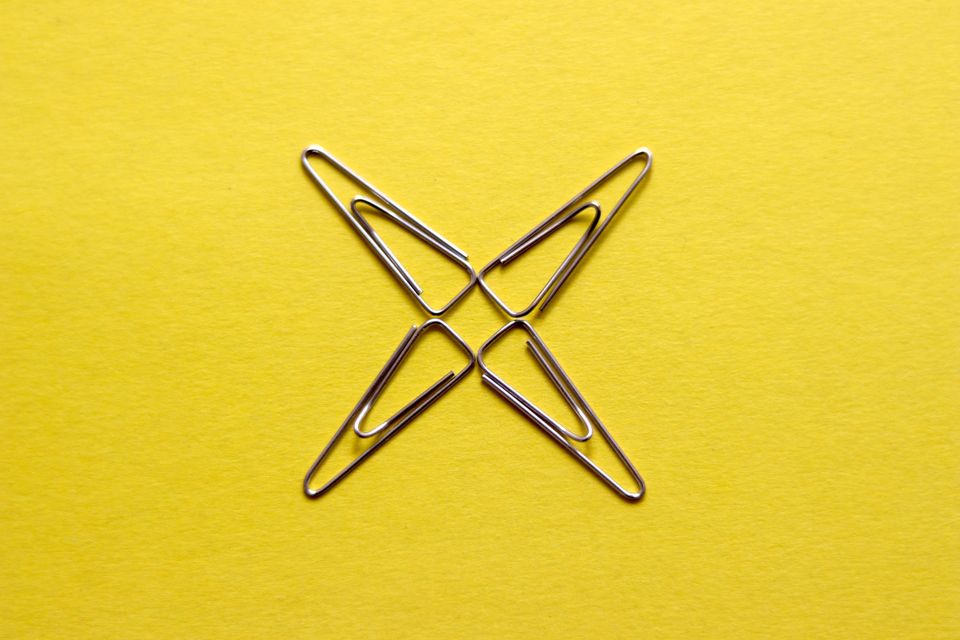
Thanks to Florian Brandl and his fellow researchers for extensive comments.
Previously I wrote about the different mechanisms for funding public goods without relying on a centralized party.
Recently, a new contender appeared on the block, arguing that the "Nash product rule" can be used to facilitate public goods production. What are the benefits? What are the downsides? And will it be possible to find a catchier name? Let's find out!
The problem at hand
For a gentle introduction into what are public goods, see the article above. In a nutshell, they are goods where it is hard for a seller to extract significant portion of the value it generates.
A problem with existing public goods mechanisms, is that they have a hard time balancing efficiency and incentive-compatibility. Let's explain.
The much heralded Quadratic Funding / Radical Liberalism system works by matching people's individual donations in a smart way. If people selfishly use the matches to donate to the projects they care about, this allows for the optimal provision of public goods (so its very efficient). As long as there is an external party who offers a matching fund, this works great. However, when asked whether any group would finance such a matching fund themselves, things get trickier. The matching fund might be used to fund lots of projects which some participants don't value at all, or are even very negative about! As a result, it might be impossible in many situations to raise an optimal matching fund through voluntary taxes.
Other schemes have similar issues:
"A simple way to ensure a Pareto efficient outcome would be to maximize utilitarian welfare: one could define an individual’s welfare as the amount of money disbursed to approved organizations and then maximize the sum of the welfare of each participating tax payer. The result would be that all the available funds would be disbursed to the (usually unique) organization that received the most “votes”. While this is efficient, it fails to provide the participation incentives of the current system: one additional vote is unlikely to change which organization is most popular, and those who do not think that this organization is worth funding will choose to not participate."
So what can we do about it?
"A result by Bogomolnaia et al. (2002) about group fairness implies that among separable social welfare functions, there is only a single candidate that might work: maximizing the Nash product, which selects the allocation of funds that maximizes the product (rather than the sum) of utilities."
The solution
Instead of using an external matching fund to "pull" people's contributions into a more optimal way, we can instead try to infer people's interests and let them re-order their contributions in a way which is mutually beneficial.
A crucial assumption to make this work, is that players have linear utility functions. To give an example: eating 2 apples will give them twice as much utility as eating 1 apple, donating 2 USD to a particular charity will give them twice as much utility as donating 1 USD.
As a result, you can mix-and-match people's donations. Let's see how such a reordering can look like in practice. Imagine two agents are asked to donate to two causes that they care about, cause A and cause B. If agent 1 donates to cause A, they get utility of 1, if agent 1 donates to cause B, they get utility of 0. For agent 2 the utilities are 1 and 3 respectively:
Utility for donating to cause A | Utility for donating to cause B | |
Agent 1 | 1 | 0 |
Agent 2 | 1 | 3 |
Let's say that both agents each have 1 USD to distribute over the two causes. The Nash product rule requires us to distribute the donations among the two causes in such a way that it maximizes the product of everyone's utilities:
MAX(c_a*(c_a + 3c_b))
whereby c_a is the total contribution to cause A and c_b is the total contribution to cause B. It turns out that this is maximised when project A receives 1.5 and project B receives 0.5.
If Agent 1 would donate 1 to cause A, and Agent 2 donates 1 to cause B, total utility would be 4. If however, the donations flow according to the Nash product rule, total utility would be 4.5!
The key innovation in this scheme is its decomposability, namely the fact that the ultimate distribution of contributions can be decomposed into distributions for individual agents, such that they spend their individual contributions only on projects which they care about. As a result, the scheme achieves a form of contribution incentive-compatibility: loosely speaking, a mechanism with this property incentivizes agents to contribute their money to the mechanism rather than spending it on an outside option (e.g., some private good).
Different types of welfare philosophies
I have actually written earlier about the the Nash welfare scheme in this blog, back for the second article The AI Economist: Improving Equality and Productivity with AI-Driven Tax Policies. What is interesting about a scheme which aims to maximize the multiplication of everyone's utilities, is that it is a kind of balance between two more popular welfare schemes:
- Maximum utility/efficiency welfare, where we simply try to maximize the sum of everyone's utilities
- Maximum egalitarian welfare, where we may try to maximize the welfare of the poorest (group of) agent(s).
Imagine people protesting on the streets one day, not demanding an egalitarian society, but a Nash product society. Well, we will need a bit of a catchier name than this. Perhaps a Considerate society, considering everyone's utility functions equally. Naming suggestions are welcome!
Bringing it into practice
Small amounts of big donors, or fully automated donor systems, might be able to adhere to the rule and accept its optimality in certain scenario's. An interesting question is whether there is any way to make the user interface more intuitive.
Similar to how quadratic matching can be shown as a curve with diminishing marginal returns, showing a preliminary estimated match, the model could show - based on other people's real or estimated donations - how the total donations attracted to your projects differs as you distribute a different amount of funds over projects. Perhaps these potential increases can be indicated as a heatmap over projects.
Combine this with a tool like mutual matching - only commit to contributing if you reach a certain level of match through the mechanism - could be very powerful as well.
The Product Nash Rule does come with a number of shortcomings. As indicated above, the mechanism (currently) assumes linear utility functions and projects which can take an arbitrary amount of funding, whilst in reality, projects might have real funding limits. Moreover, more analysis will have to be done to see how the mechanism can be made strategy-proof. the Nash rule is manipulable in the sense that agents may have an incentive to report utilities different from their true utilities. Also, we prove our results only for linear utility functions and projects that can take an arbitrary amount of funding.
In Vitalik Buterin's most recent analysis of retrospective public goods funding, he makes the case that testing for funding limits might be less of an issue compared to prospective public goods funding. Therefore the Product Nash Rule might be best suited in such a context.
To get an idea of how the Product Nash Rule leads to different allocations compared to the "maximization of efficiency", check out this awesome tool from one of the authors of the Nash Product Rule: https://dominik-peters.de/demos/portioning