The AI Economist: Improving Equality and Productivity with AI-Driven Tax Policies
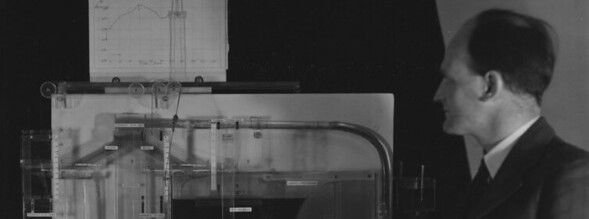
Today let’s talk about one of two things you can never avoid in life: taxes! [1] Unfortunately, today’s paper can’t help you determine taxes or file your tax declaration, but it does aim to make them a little bit more fair:
In this work, we train social planners that discover tax policies in dynamic economies that can effectively trade-off economic equality and productivity. We propose a two-level deep reinforcement learning approach to learn dynamic tax policies
The AI Economist is trained to learn an optimal tax schedule, indicating which income brackets are supposed to be taxed by which tax rates. Many countries have a progressive tax system whereby people earning higher incomes have to pay more taxes, but the question remains how much they should be taxed.
The first things which the AI Economist reminded me of were the early simulations of inventor Bill Phillips, who used the flow of water to model the economy:
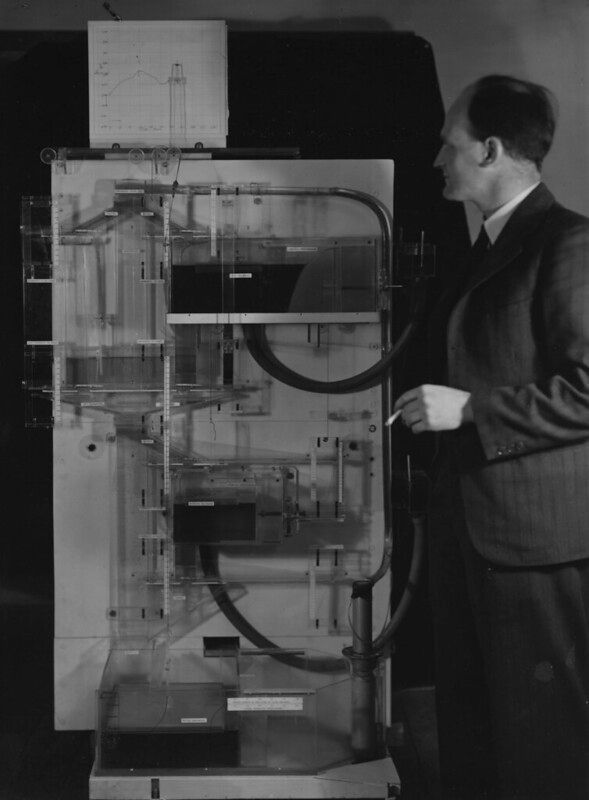
However, in the age of computers, we don’t need water! Instead, we’re using an artificial Gather-and-Build game where simulated agents exchange digital coins:
Agents move around, collect resources (wood and stone) and build houses. Agents cannot move through each others’ houses, or move through water. Agents can trade resources.
In the simulation the profit-maximizing agents soon learn to specialize, given that they are endowed with different levels of skill and environmental benefits. It is this specialization which also drives inequality:
The dark- and light-blue agents focus entirely on collecting wood and stone (respectively), the orange agent focuses almost entirely on building houses, and the yellow agent builds several houses early on before switching to collecting and selling
Economical (and philosophical!) background
Many governments like to tax people in order to (among other goals) redistribute income. This is easier said than done. Some of you may be familiar with the Laffer curve, which makes the logical case that when you tax 100% of people’s income, you are probably not going to necessarily raise more taxes:
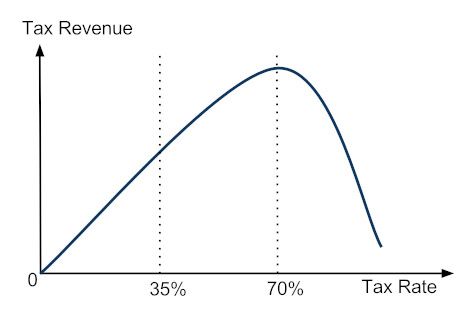
The core challenge in the design of optimal tax policies is that taxes and transfers can affect incentives to work, creating a trade-off between equality and productivity [Mankiw et al., 2009, Diamond and Saez, 2011]. A particular concern is that high income may correlate with high skill, leading higher skilled workers to choose to work less.
So how should we trade-off equality and productivity then? This is a fundamental political question, one which has taken center stage in democratic elections for as long as I can remember. A related philosophical tradeoff exists between the values of equality versus freedom: a predictable way to increase people’s equality is to curb their freedom and to tax their assets by force.
The authors take a simple and perhaps brilliant way out: just optimize both values! They define a Social Welfare Function as the product of equality (as measured by the gini coefficient) and productivity (as measured by total income). Alternatively, Social Welfare Functions can be made which place a higher value on the preferences of the poorest, or where the payments made by an individual merely match the benefits received. As a base scenario we can use:

The results in this paper are compared to those achieved by the Saez tax framework which:
derives optimal income tax formulas using compensated and uncompensated elasticities of earnings with respect to tax rates. A simple formula for the high income optimal tax rate is obtained as a function of these elasticities and the thickness of the top tail of the income distribution.
However, the AI Economist (I’m starting to like the name) can manage a better trade-off:
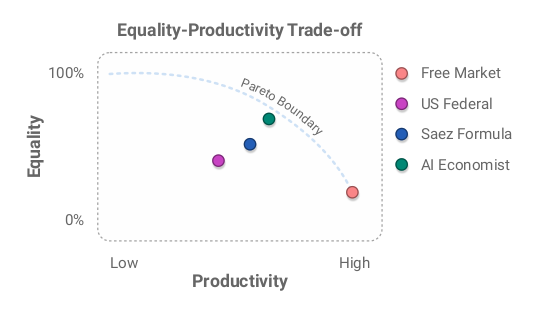
Machine Learning background
In the AI Economist’s two-level deep reinforcement learning framework, agents maximize their selfish expected returns while tax policy officials maximize social welfare. The framework has the following technical characteristics:
- A particular challenge presented by the AI Economist is that it presents a two-level learning problem, in which the social planner learns a tax policy simultaneously with agents who learn how to optimize their behavior.
- In the present paper, we insist on each agent having a policy that only makes use of information that it can individually observe.
- Formally, we build on the framework of partial-observable multi-agent Markov Games (MGs) (a fancy term for agents having a probabilistically updating state, one day they’re rich, the other day they are poor, etc.).
- Spatial observations are processed by a stack of two convolutional layers (CNN) and fattened into a fixed-length feature vector. This feature vector is concatenated with the remaining observation inputs and the result is processed by a stack of two fully connected layers (MLP). The output is then used to update the hidden state of an LSTM and action logits are computed via a linear projection of the updated hidden state. Finally, the network computes a softmax probability layer for each action head.
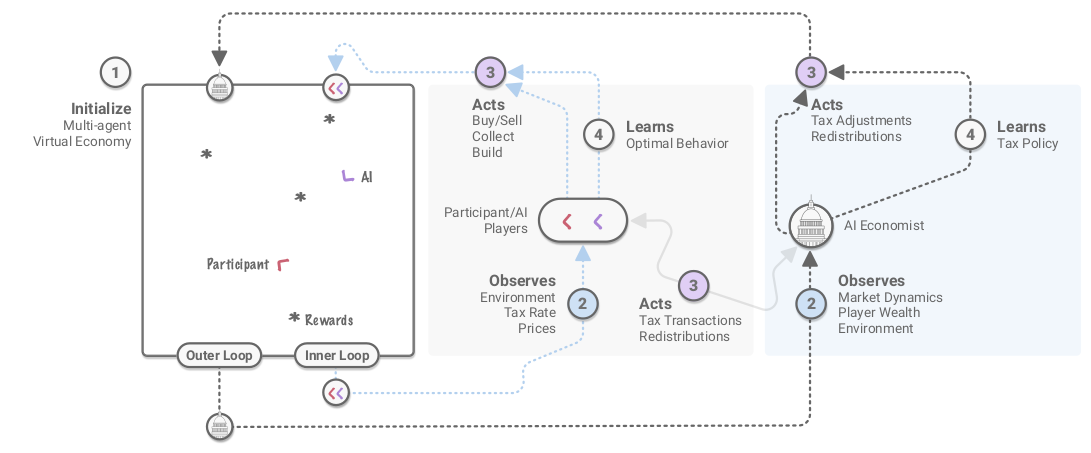
As a result of all this magic, the AI Economist manages to:
improve the trade-of between equality and productivity by 16% over baseline policies, including the prominent Saez tax framework [...] Under the Saez scheme, the “buyer-and-builder” collects more resources directly from the environment, meaning it makes fewer purchases from the other agents. [...] We see emergent tax gaming, where AI agents learn to lower their average effective tax by alternating between earning high and low incomes in each period, rather than smoothing their income across tax periods
I believe much is left to discover with this new approach. As the framework is open source the hurdle shouldn’t be big for aspiring economic computer scientists to develop their own frameworks. This framework allows us to rapidly experiment and play with all parameters, which may lead to more correct results in general:
In the place of experimentation, economic theory often relies on simplifying assumptions that are hard to validate, for example about people’s sensitivity to taxes.
Caveats and further improvements
Time for some expectation management. Although the results of the paper are exciting, there are a number of caveats to keep in mind.
In a democratic society it is essential that legislation is understandable and legible. Machine learning models are some of the most enigmatic systems out there, the contents of which are classified as black boxes. In other words, we don’t always understand why machine neural networks decide what they do. Besides being hard to understand for decision makers, complex models also risk being overly optimized and overfitted to a particular situation. A potential way out is to take the concept of Automated Mechanism Design to its extreme and to create a higher number of highly optimized policies for different situations.
Moreover, we may not only want to set tax levels to maximize productivity and equality. Although the U.S. government is not achieving an optimal trade-off between equality and productivity according to this paper, the U.S. tax brackets were shaped by countless other factors such as (1) requiring taxes to subsidize certain sectors (2) the influence of other taxes and (3) practical constraints. To give an example of the latter: the Dutch tax authority recently asked their parliament to stop making new rules because their IT systems couldn’t handle the changes. [2]
And of course, the biggest problem in making any coordination mechanism work are the humans it tries to coordinate:
we have observed that humans display a higher frequency of adversarial behavior, such as blocking other people. These kinds of behaviors are socially suboptimal, but might seem optimal to people (keeping resources to oneself). This can be partially attributed to a lack of trading, but also hints at a common human intuition that blocking of regions with resources should be an effective strategy
Footnotes
[1] Given that the second thing you can’t avoid in life is death, perhaps Equilibria Club should review a paper on the pricing of funeral services.
[2] I think some people didn’t manage to stay DRY
For a visual tour of the paper, check out: https://www.youtube.com/watch?reload=9&v=Sr2ga3BBMTc